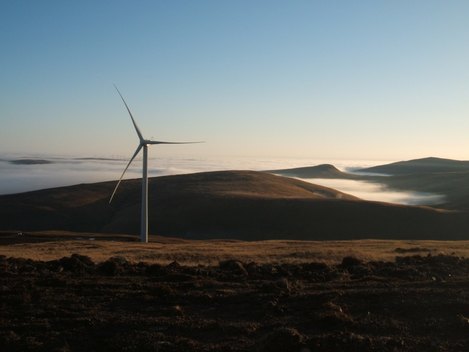
March 22, 2021, by Chris Handley
CITRUS – Smarter Power
Changing Times
How we are using energy is changing. Not only how the energy is generated, but where and when it is used. These changes mean that we are now pushing the limits of how we expected to use our traditional gas-fired power stations, both in terms of the longevity of these sites, the operation of the sites, and how we can deliver energy without a break in supply. The ultimate goal is to address these issues in a manner that leads to greater sustainability.
Power generation once relied on how society operated — a typical 9 to 5 day, with a surge in power demand during the ad breaks of certain soap operas. But the modern, digitally-enabled world, means a disruption in how, when, and where people work, which requires power generation to respond more dynamically beyond the traditional operational expectations. This introduces stresses into the power station components which have not been previously modelled, leading to a component breakdown.
But what if we could model the breakdown, and predict when a component will break?
That is the goal of the work of the DRS as we support the research of Dr Chris Hyde and Dr James Rouse of the School of Engineering, and their collaboration with EDF Energy. CITRUS — Component Integrity Tracking and Readiness Utilization System.
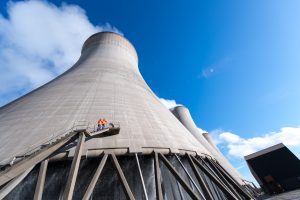
West Burton A coal power station
We have the Data
Power stations utilise an “internet of things”, with sensors located near points of interest on the components — typically wear fractures and breakdown most likely occurs — relaying real-time information regarding the local temperature of the component. And it is from that we can begin to predict the stresses being induced – and from that predict the wear and tear on a component.
Stress in a component can be modelled using Finite Element Modelling, for any component geometry, temperature change, and material. But to model, responsively, the stresses as temperature varies on a series of different components, cycling up and down during a period of operation, is an intractable task. However, what if we used Machine Learning?
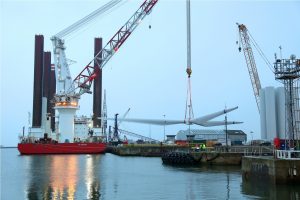
Teesside offshore wind farm – rotor blades lift 02
Emulation not Simulation
Machine Learning means using algorithms to learn patterns within data so that it can make predictions for unseen cases. The Machine Learning algorithm learns the non-linear relationship between inputs and target outputs from the data sample provided, enabling it to make interpolations and predictions for any set of inputs within the feature space the algorithm has been trained for.
The Machine Learning method implemented is neural networks. Taking the descriptors regarding the component and the temperature, as inputs, the outputs predicted are the three characteristic values that describe the stress response due to a 1-degree change in temperature. In effect, the neural networks, trained on the data provided by FEM simulations, allows for the emulation of FEM but for the fraction of the compute cost. Now in a fraction of the time, the stress response of a component can be modelled for an entire day’s worth of operation.
Furthermore, this approach opens up new options in station component design – proposed modes of operation, or proposed component designs can be rapidly evaluated to see what stresses are induced, and thus which configurations lead to longer usage lifetimes. Being able to predict when a device needs to be replaced means it can be done in a controlled manner, with little disruption to power generation, or detect when the sensors on components start to drift and return erroneous data that would lead to unrequired shutdowns.
This is only the beginning for CITRUS as the DRS has helped realise an initial version of the software. The implications of the method and software to adjacent problems are significant, such as green energy generation, the prediction of the breakdown of components on high-performance vehicles, or the prediction of wear and tear on machines used in extreme environments such as mining.
No comments yet, fill out a comment to be the first
Leave a Reply