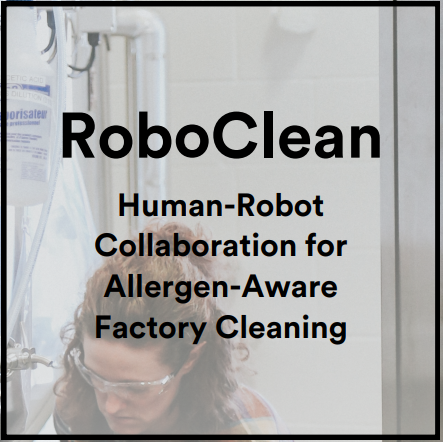
May 4, 2020, by Hazel Sayers
RoboClean
RoboClean – a Beacon Demonstrator project on Natural Language in Human-Robot Interaction and Allergen Sensing
In food and drink manufacturing, cleaning takes a significant amount of employee time, bearing a major impact on productivity and manufacturing efficiency. One of the biggest challenges faced by manufacturers is the cross contamination of allergens. The RoboClean project has explored the two-fold challenge of a) natural-language interaction with service robots – robotic vacuum cleaners – as a proxy to investigate effective collaborative robotics in factories, and b) allergen sensing in powdered foods using near-infrared spectroscopy. The vision is that future work will combine the two strands of work into one hardware platform, enabling collaborative cleaning bots that scan for allergens while cleaning the floor.
In terms of the two strands of work, the project has made significant steps to bring together the work on human-robot interaction with the work on allergen sensing, and data visualisation, in what may be called the RoboClean process to make socio-technical co-bot systems (see Fig. 1).
Fig 1.
Fig. 1. The RoboClean process.
The process captures the stages that would be needed to create bespoke co-bot systems, by example of robovacs for specialised environments such as food factories.
First, the project commences with site visits, which are needed for the robot to map the space, and to learn from the workers the locally specific practices, names and terminology for objects and areas within the space, cleaning schedules, priority areas, deadlines (e.g., by when does the area need to be cleaned?), which kinds of allergens are expected, and so on. This is needed to create bespoke cleaning zones with labels (i.e., names) that are meaningful and intuitive to those eventually instructing the cleaning robots by voice. We have conducted an elicitation study in a university Engineering laboratory to elicit the language people use, and further explore machine learning techniques to make use of the resulting data corpus to build language models for voice control in specialised environments (publication forthcoming).
Second, the information on cleaning areas, priorities, deadlines, and so on can then be used to bootstrap multi-agent coordination for task allocation. In a setting with multiple robots, this can be used not just to simulate the most effective schedule of the cleaning which can be useful for communicating and planning, but to build a partially automated, partially human-controlled multi-robot system (publication forthcoming).
Third, building on the insights gathered in the field and in the simulation (in step 1 and 2), an app to control the robovacs by voice – including bespoke names for certain areas in the space – can now be deployed. We built a protoype Amazon Alexa app that allows just that. There is also a fully working backend system that connects to the Neato API to allow for controlling multiple Neatos (the robovacs we’re using in the project).
Fourth, work to classify powdered foods (a proxy for certain allergens) based on near-infrared spectroscopy has been published in the Sensors journal [Rady et al., 2020]. The work showed high accuracy for the classifications of different powdered foods, and makes recommendations for optimal sensor height and light intensity. Forthcoming work looks at accuracy under mobile conditions, to approximate the performance of a robot-mounted sensor.
Fifth, for auditing purposes the sensor data readings can be made available via a data visualisation platform. In the project we explored the use of Intelligent Plant’s app store for this purpose.
Overall, the RoboClean project demonstrates a “proof-of-concept” in bringing together multiple challenging areas in disciplines including Engineering and Computer Science, including Human-Robot Interaction, Natural Language Processing, Data Science, and Human-Centred Design.
REFERENCES
Rady, A.; Fischer, J.; Reeves, S.; Logan, B.; James Watson, N. The Effect of Light Intensity, Sensor Height, and Spectral Pre-Processing Methods When Using NIR Spectroscopy to Identify Different Allergen-Containing Powdered Foods. Sensors 2020, 20, 230.
No comments yet, fill out a comment to be the first
Leave a Reply