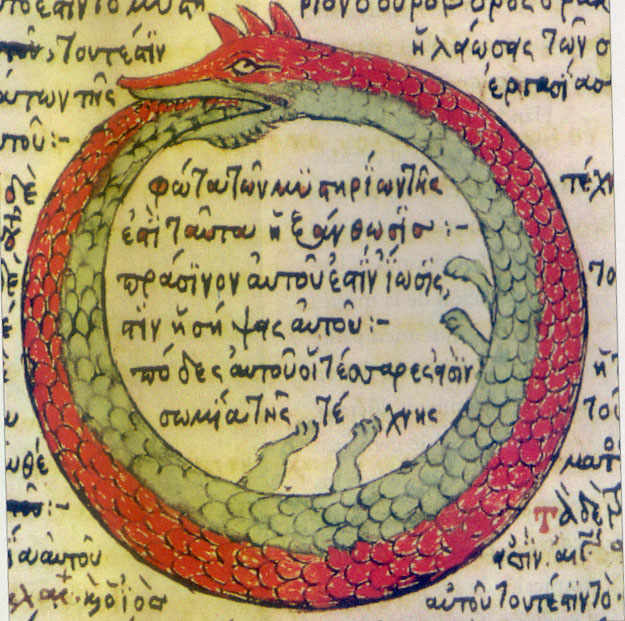
April 19, 2024, by Brigitte Nerlich
From contamination to collapse: On the trail of a new AI metaphor
I wrote my first ever post about AI and ChatGPT on 6 January 2023. Amongst other things, I talked about the danger of ‘knowledge pollution’. I wanted to highlight the dangers of a gradual corruption of our knowledge base.
Knowledge pollution
ChatGPT and many other bots or AIs like it are based on large language models or LLMs. LLMs retrieve, rearrange and synthesise existing human knowledge and information sucked up mainly from the internet. Under normal circumstances, existing human knowledge increases over time and gets more reliable over time. However, that rising pool of knowledge will increasingly be fed by the outputs of ChatGPT and other bots. Some of that output is far from accurate or correct, indeed, some say much of it is bullshit. And so, over time, our pool of knowledge may gradually get polluted and diluted. That’s what I referred to ‘knowledge pollution’.
In my most recent post about AI, published on 12 April 2024, I tried to collect a number of AI metaphors, amongst them pollution and contamination metaphors which have begun to spread quite widely. These pollution metaphors are based on mapping similarities between the pollution of ecosystems and the pollution of knowledge systems.
While I was gathering examples of pollution and contamination metaphors for last week’s post, another metaphor was lurking in the background that I didn’t see, namely the metaphor of ‘model collapse’, sometimes also referred to as ‘knowledge collapse’ or ‘AI collapse’. (And like with the metaphor of knowledge pollution, I have to thank my son for alerting me to that metaphor!)
In this post I want to chart the beginnings of this new AI discourse centred around a new metaphor. As usual, I stress that I don’t really understand AI and so you have to take this with a pinch of salt.
Model collapse
The new discourse of ‘model collapse’ began to spread in mid-2023, it seems; and the new metaphor at the centre of it was more surprising than I thought. I had assumed that just as the pollution metaphor maps what we know of air or water pollution onto knowledge and the internet, so the collapse metaphor might map what we know about the disintegration of buildings or ecosystems onto LLMs and knowledge. But, when I googled ‘model collapse’, it became clear that the metaphor is also anchored (probably via the contamination metaphor) in another conceptual domain, namely disease.
According to a short Wikipedia article, ‘model collapse’ refers “to the gradual degradation in the output of a generative artificial intelligence model trained on synthetic data, meaning the outputs of another model (including prior versions of itself).” That was interesting. Whereas I had focused in my first blog on the pollution of ‘human’ knowledge, this metaphor focuses on the destruction of ‘artificial’ knowledge, i.e. (generative artificial intelligence) models.
So, models are said to collapse when they ‘feed’ on their own output. This goes beyond mere pollution in the sense of an oil spill, I thought, and is more like mad cow disease (where cows are fed ground up cows or sheep), or the recent outbreak of bird flu in cows (where cows are fed ground-up chicken), or the fictional Soylent Green scenario (where humans are fed ground up humans). What an analogy!
Mad AI disease
The wiki article on ‘model collapse’ explains: “Repeating this process [of training models on their own output] for generation after generation of models forms a so-called autophagous (self-consuming) loop.” And there it comes: “Theoretical and empirical analysis has demonstrated that, without enough fresh real data in each generation of an autophagous loop, future generative models are doomed to have their quality (precision) or diversity (recall) progressively decrease. Hence, model collapse has also been termed Model Autophagy Disorder (MAD), making an analogy to mad cow disease” (bold highlighting removed); some even call it AI prion disease.
BSE or mad cow disease (a prion disease) came about through feeding cattle “meat-and-bone meal (MBM) that contained either the remains of cattle who spontaneously developed the disease or scrapie-infected sheep products.”
The paper on which this wiki article is based was published in mid-2023 – here is a longer summary. The authors of that paper quote another important article from 7 March 2023 (revised 14 April 2024) in which another group of researchers describe what they call in a nicely recursive way “The Curse of Recursion”. The main contention of the article is that too much synthetic data fed into models causes “model collapse” and makes the models “more prone to just focusing on probable results and less likely to produce interesting but rare results”.
The authors have some recommendations about how to avoid total collapse though. They recommend basically to not just scrape data from the internet (which strangely reminded me of scrapie), but instead to also collect (fresh) data about “genuine human interactions”. Another paper, published on 1 April 2024, also argues that model collapse is not inevitable.
But the dangers of model collapse, based on MAD or, as some say, using a slightly different metaphor, AI inbreeding, are still quite big I think, and might not only lead to widespread knowledge collapse but also to a debasement of expert or specialist knowledge. Everything will become beige….
Knowledge collapse
Knowledge, rather than model, collapse is discussed in a paper by Andrew J. Peterson published quite recently in April 2024. He warns that “overreliance on AI-generated content could lead to a phenomenon he terms ‘knowledge collapse’ – a progressive narrowing of the information available to humans and a concomitant narrowing of perceived value in seeking out diverse knowledge.”
As Maximilian Schreiner says in a blog post from which I just quoted: “Widespread recursive use of AI systems to access information could therefore lead to the neglect of rare, specialized, and unorthodox ideas in favor of an increasingly narrow set of popular viewpoints. This is not just a loss of knowledge – the effect also limits the ‘epistemic horizon,’ which Peterson defines as the amount of knowledge that a community of people considers practically possible and worth knowing.”
Conclusion
Here we are back to the issue of pollution, not so much of the atmosphere but of what I called in last week’s post the epis-sphere, the sphere of human knowledge. Some people actually wonder whether “AI could choke on its own exhaust as it fills the web”….
Despite the fact that people are working to avoid model, knowledge or AI collapse, the prospect of this happening is quite alarming. I wonder how this type of worry compares with the existential risk discourse of civilisation collapse, where AI doesn’t only feed on itself but also feeds on us. What is hype and what is reality?
And may there be a link between the two, between model collapse and civilisation collapse? Or is that even worse hype? Probably. But…as Stephan Lewandowsky and a team of other experts have argued quite recently, a functioning democracy relies on citizens sharing a reliable and trusted body of knowledge. Knowledge pollution and model collapse might erode this foundation of democracy.
Addendum: Nora Lindemann has just published an interesting article on increasingly ‘sealed knowledge’ which resonates with this post.
Image: Public domain: An ouroboros in a 1478 drawing in an alchemical tract
Previous Post
Hunting for AI metaphorsNo comments yet, fill out a comment to be the first
Leave a Reply