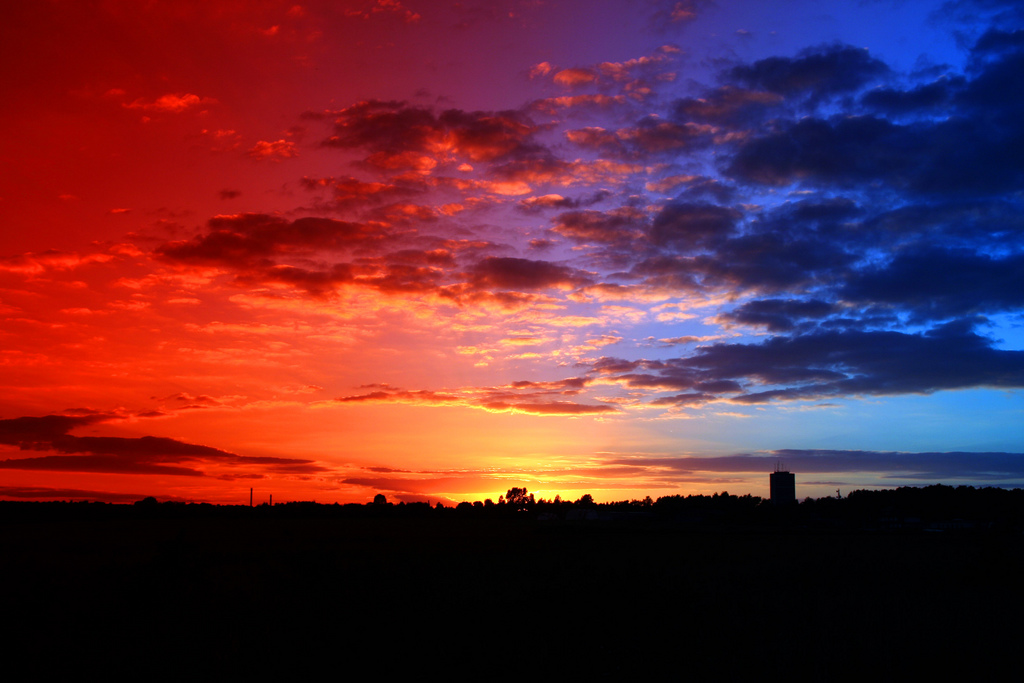
August 25, 2023, by Brigitte Nerlich
Red and blue AI?
This is another post about artificial intelligence or AI, but it’s what one may call a bit ‘experimental’. I happened to think about an analogy and ran with it, but it might be a completely inappropriate one. Let me know!
Red and green GM
About twenty years ago, at the turn of the millennium, I helped to establish the “Institute for the Study of Genetics, Biorisks and Society” later called the Institute for Science and Society. That was the time when controversies around genetic modification, cloning, BSE and so on dominated the headlines. I remember us thinking about the difference in coverage of and attitudes to what some called ‘red’ and ‘green’ GM, that is, genetic modification for medicine and genetic modification for food and crops. In 2002 Martin Bauer talked about the emerging “contrast between ‘desirable’ biomedical (RED) and ‘undesirable’ agri-food (GREEN) biotechnology”.
If we were thinking of setting up an institute nowadays, we would probable call it something like the “Institute for the Study of AI, Cyber-risks and Society” and perhaps we would be thinking about the emerging contrast between red and green AI or perhaps better red and blue AI. What do I mean by that?
Promising or polluting AI
This distinction came to me when listening to two announcements about advancements in medical AI yesterday, advancement which seem to be coming strong and fast. First I heard about the possibility of determining someone’s heart age as compared to someone’s actual age. Then I heard about the possibility of predicting the risk of Parkinson’s disease from looking at various layers in the retina and analysing biomarkers. Both these advances are related to medical image analysis, as were previous advances in radiology using AI for the detection of breast cancer and so on. In general, the use of algorithms and AI in healthcare (RED AI) seems to be reported in quite a positive light.
At the same time. huge advances are being made in AI using large language models to produce what is called ‘synthetic text’ or help in coding, and here the situation seems to be quite different, as dangers are being discussed from hyped-up existential threats to more tangible dangers, such as discrimination, automated criminalisation and more. This type of AI, or generative AI, is reported in more negative ways, it seems (BLUE AI).
One of the biggest problems with generative AI is perhaps the pollution of knowledge and truth and trust by, as John Thornhill points out, “adding more imperfect information and deliberate disinformation to our knowledge base, […] producing a further ‘enshittification’ of the internet, to use Cory Doctorow’s evocative term”.
I talked about this in a blog post from 6 January this year (and this includes a great summary of the possible pollution of knowledge by ChatGPT itself!). Others have, of course, also noted this problem using interesting metaphors.
Emily Bender, a professor of linguistics, talks for example about the creation of “an equivalent of an oil spill into our information ecosystem”. In a thread on Mastodon Rich Felker also taps into the dangers posed by fossil fuels to frame the dangers of AI: “AI is a lot like fossil fuel industry. Seizing and burning something (in this case, the internet, and more broadly, written-down human knowledge) that was built up over a long time much faster than it could ever be replenished.” Both critics of AI draw inspiration from the dangers posed by the fossil fuel industry and climate change, something I can’t see when people discuss AI in healthcare.
Red and blue AI – really?
So, is there a difference between red and blue AI? I am not totally sure yet.
When asked about the benefits of AI, many people might say something like ‘diagnosing diseases’ and when asked about the risks of AI they might say bullshit, confabulation and disinformation. But is that enough to think there is an emerging dichotomy between red and blue, good and bad?
In both cases, there are dangers to workers in various industries, algorithmic discrimination and so on. In both cases, the medical/imaging advances and the generative/large language model advances, we are dealing with a process of pattern matching. So shouldn’t that lead to the same issues, problems and risks? Regarding, for example data extraction? Where do the data, images or words come from, who has provided them with or without consent?
And where do robots and autonomous vehicles fit in? I don’t know…..
However, if there is at least a slight perceived difference between what I call red and blue AI, that is, medical AI and non-medical AI, could this have impacts on people’s attitudes to ‘AI’? Would they see medical or red AI as “useful, morally sound, and to be encouraged”, as Bauer found with red GM (2002, p. 97), while they might be more sceptical about non-medical or blue AI? Again, I don’t know. But I think it is something worth thinking about in the context of researching responsible AI or trustworthy AI. More research needed!
Image: Red Blue Sunset (Wikimedia Commons)
AI that depends on trawling big datasets.. is only going to be as good as the information on the dataset.. and is possibly just a category error.. Tesla’s approach for example..
additionally I was discussing this with an AI consultant a few weeks ago. they had a project looking at parkinsonism, dementia, etc and were working with medical professionals, and even their there was not just quality data issues. but a big issue was the subjective nature of the data. ie
too be honest a lot of the recent AI hype, cones across in a similar vein as how computer ‘expert systems’ were going to replace people, from over 20 years ago.
there are also lots of risks I think with AI. but not so much as it will be do intelligent and will harm people.. but in reality it is so ‘dumb’ but is deployed and will harm people. think Autonmous cars or armed drones.
Professor Mark Bishop (one of my former lecturers) has some good papers on this. He has been arguing the dumb AI case vs the AI are going to be taking over the world case (Prof Kevin Warwick, Hawkins, etc) for over 30 years. Kevin also lectured me. Head of Cybernetics department.
Finally a topic I am ‘qualified on’ lol.
My supervisor had one of the very 1st Phd’s in the field of Virtual Reality. and my thesis was modelling for department VR system use. And importantly the philosophy of AI.
A lot of debate now is very, very similar to the last 10,20,30 years and we have a new cycle of hype.. Not to discount AI and the impact it could have.. but not the Hollywood layperson expectation
Yes, it all feels very new and also very old at the same time. I remember the expert system times, the Kevin Warwick times, and so on. I really wonder how it all will play out.
Artificial Intelligence Is Stupid and Causal Reasoning Will Not Fix It JM Bishop Frontiers in Psychology
Department of Computing, Goldsmiths, University of London, London, United Kingdom
https://www.frontiersin.org/articles/10.3389/fpsyg.2020.513474/full
quote.
“we need to stop building computer systems that merely get better and better at detecting statistical patterns in data sets—often using an approach known as ‘Deep Learning’—and start building computer systems that from the moment of their assembly innately grasp three basic concepts: time, space, and causality.” In this paper, foregrounding what in 1949 Gilbert Ryle termed “a category mistake”, I will offer an alternative explanation for AI errors; it is not so much that AI machinery cannot “grasp” causality, but that AI machinery (qua computation) cannot understand anything at all”
https://m.youtube.com/watch?v=e1M41otUtNg
this is worth a watch.. Lecture and discussion about this
and another (shorter) paper
The Singularity, or how I learned how to stop worrying and love AI
https://core.ac.uk/download/pdf/29132477.pdf
https://m.youtube.com/watch?v=e1M41otUtNg
this is worth a watch.. Lecture and discussion about this
and another (shorter) paper
The Singularity, or how I learned how to stop worrying and love AI
https://core.ac.uk/download/pdf/29132477.pdf