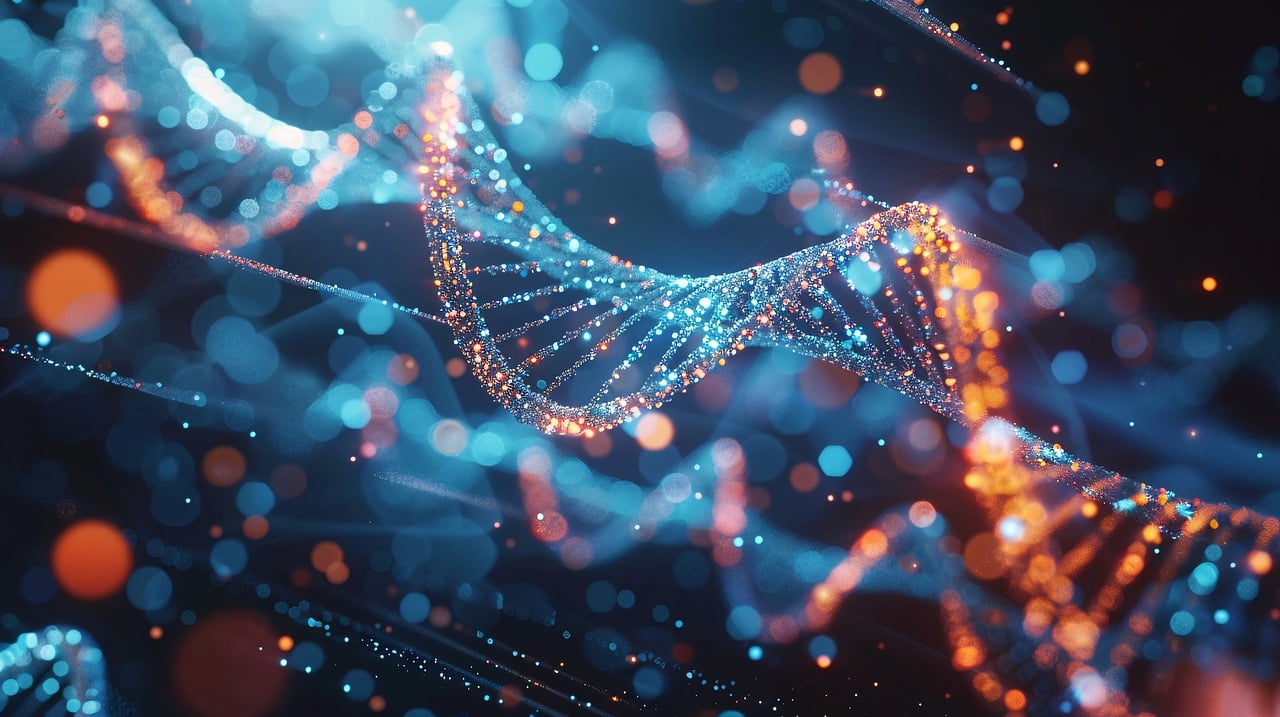
August 9, 2024, by Brigitte Nerlich
From large language models to DNA language models
In October 2023 I wrote a blog post about a convergence of large language models or LLMs and DNA. LLMs are subset of generative AI that focus on generating and understanding human language and producing human-like text. DNA is often compared to a language or a code.
In the post I quoted a representative of Ginkgo Bioworks as saying: “The same way that foundation models like OpenAI’s GPT are trained on the English language, they can ‘learn to speak DNA (…) DNA is a sequential coded language, very similar to a book.’” A lot has happened since then, some of it captured in this blog post by Jim Thomas on ‘DNAI’ published in January 2024.
I was just thinking about all this fast moving stuff, when I came across a press release in which one of the main authors of a new paper is quoted as saying that they have developed an AI language model that “has basically learned how to ‘speak’ DNA”. Is that just hype, I wondered…? At closer inspection this paper seemed different to previous ones. Why?
Language and ambiguity
When we first started to speak, or rather to speak about, the ‘language of life’ (or reading the book of life or cracking the code of life), the philosopher of science, Lily E. Kay wrote in her seminal book Who Wrote the Book of Life? A History of the Genetic Code (2000) that DNA cannot be readily compared to any natural language because “it lacks phonemic features, semantics [meaning], punctuation marks and inter-symbol restrictions” (Kay 2000, p. 2). She claims that once “the genetic, cellular, organismic, and environmental complexities of DNA’s context-dependence are taken into account [genetic messages…] read less like an instruction manual and more like poetry, in all their exquisite polysemy [multiplicity of meaning], ambiguity, and biological nuances” (pp. xviii-xix).
Having worked on ambiguity and polysemy in natural language myself, this quote has always stuck in my mind and reminded me of the shortcomings of the ubiquitous language, book, code etc. metaphors that pervade genetics and genomics. This does not mean that these metaphors are useless. Far from it. They have guided researchers to many insights into how genes and genomes work.
Anyway, when I read the press release for the AI model that has learned to speak DNA, this quote came back to me and I wondered how this new paper dealt with the issues highlighted by Kay.
A new DNA language model
A team of researchers at the Biotechnology Center (BIOTEC) of Dresden University of Technology in Germany (Melissa Sanabria, Jonas Hirsch, Pierre M. Joubert and Anna R. Poetsch) developed a deep-learning model, also called a DNA language model or DLM, named GROVER (Genome Rules Obtained Via Extracted Representations). They trained it on human DNA and designed it to understand and perform tasks related to the human genome by treating DNA sequences much as how natural language is processed in natural language processing models.
Attempts to analyse DNA sequences as ‘text’ are based on a deep-rooted analogy between natural and biological language. DNA is composed of four basic chemical ‘building blocks’ called nucleotides, often referred to by their single-letter abbreviations: Adenine (A), Thymine (T), Cytosine (C) and Guanine (G). These are called the ‘letters’ that make up DNA. A goes with T, and G goes with C to form chemical bonds called base pairs, which connect the two DNA strands.
From these ‘letters’, the story extends to reading or writing words/genes, chapters/chromosomes and books/genomes. This story is now regarded as rather simplistic, especially since we now know, and the researchers stress this, that “the information hidden in the DNA is multi-layered”, that “only 1-2 % of the genome consists of genes, the sequences that code for proteins” and that “DNA has many functions beyond coding for proteins”.
The researchers also emphasise that, unlike a natural language, DNA has no defined words and “there are no predefined sequences of different lengths that combine to build genes or other meaningful sequences.” So, how to find them? That’s where AI comes in.
By what I can only regard as magic AI wizardry, GROVER learns to discern words, or rather tokens, then patterns or fundamental rules and structures of genomic sequences in context. Based on this, it extracts biologically meaningful or functional units, such as gene promoters and protein binding sites. It can also find “some epigenetic information, enhancing understanding of DNA’s non-coding regions”. Interestingly, it gets to grips with multiple layers of information encoded in the human genome, beyond just the protein-coding sequences. It even “learns context and lexical ambiguity”, it seems.
In the end, the authors want to use AI to help them “compose a grammar book for the code of life”.
So, it is claimed that GROVER not only learns the context in which sequences appear and is said to be able to handle token ambiguity, akin to understanding different meanings of words based on context in natural language, it is also expected to reveal the grammar book of the code of life. These are bold claims. I leave it to those more expert in the field to assess them.
This advance based on treating DNA as a language or a text would, I suppose, have surprised Lily E. Kay in the way it tries to deal with some of the complexities she mentioned in her book. I would just love to know a bit more about what the researchers understand by grammar in the context of DNA.
Colliding codes
Interestingly, this article appeared at the same time that Kevin Mitchell and Nick Cheney published a paper detailing the idea that “our genome is like a generative AI model”, not like a blueprint, code, programme or an instruction book. Mitchell and Cheney’s article was submitted as a preprint on 22 July, while the article by the Dresden team was published in Nature Machine Intelligence on 23 July. One article frames the genomic code as an AI, while the other uses AI to ‘decode’ the genomic code. That’s an interesting collision/convergence of ideas.
Image: Pixabay
No comments yet, fill out a comment to be the first
Leave a Reply