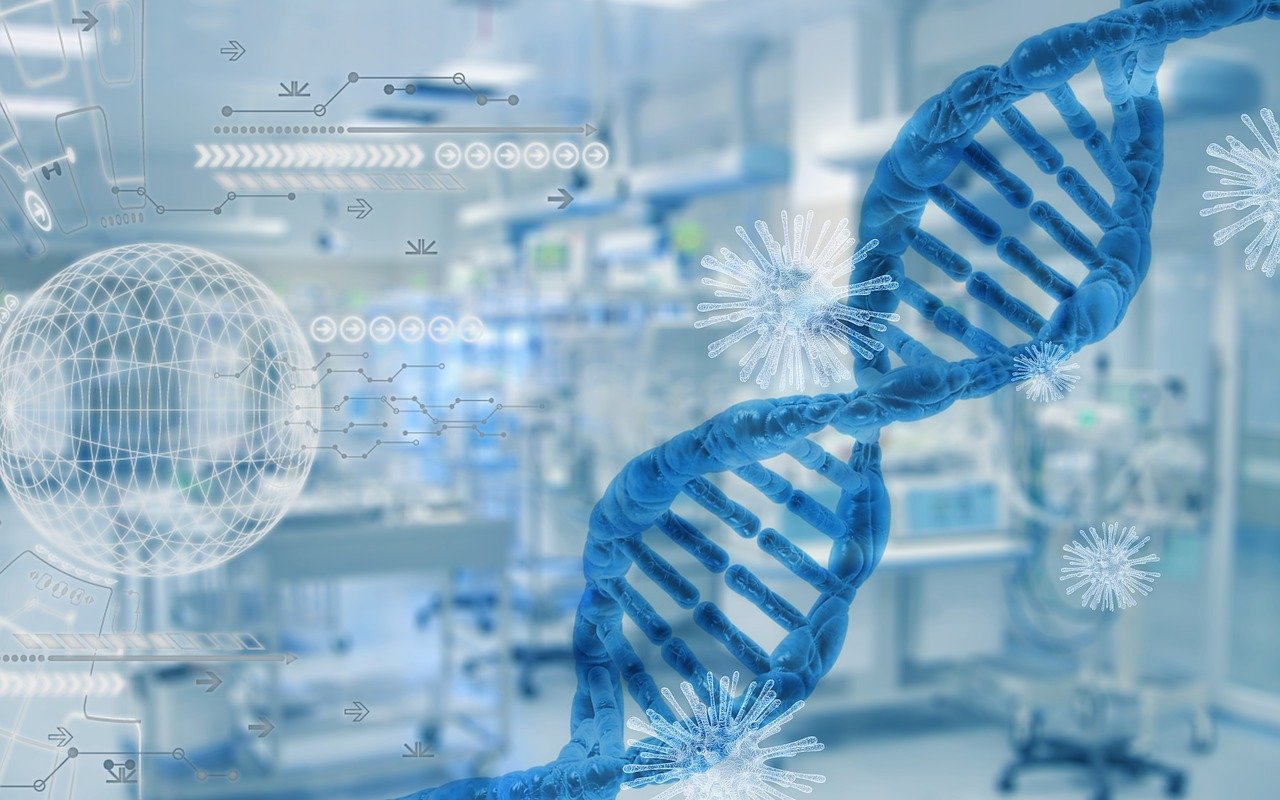
October 6, 2023, by Brigitte Nerlich
The language of life meets large language models
Between about 2014 and 2018 I was involved in the social and communications side of ‘synthetic biology’ as part of the Synthetic Biology Research Centre (SBRC) here at the University of Nottingham, which uses engineering biology approaches to understand and then modify industrially-relevant bacteria. I wrote my last blog post on synthetic biology in 2020.
That all seems a long time ago. Reading an article in The Wall Street Journal about synbio, (generative) AI, machine learning and large language models, I realised that a lot has happened over the last few years and a lot is happening now. I should at least take note of that. So, I looked a bit randomly at some news items and found some interesting shifts in language and metaphor.
I’ll first present a background case study in synbio and AI that I stumbled upon when reading the article in the WSJ, then I’ll explore some interesting metaphor developments.
Synbio and AI – a case study
When I was still involved with the SBRC, I had the privilege of meeting some people from the company LanzaTech (Dr Sean Simpson, in particular), who, like the SBRC, are deeply into carbon recycling technology So I was pleasantly surprised to see LanzaTech mentioned in the WSJ article. According to the WSJ, the company has been “using recycled carbon emissions to help create materials that can be turned into clothing, plastics, jet fuel and even perfume.” But things have changed since I last saw Sean in around 2018. As James Daniell, LanzaTech’s vice president of AI and computational biology, told the WSJ: “We’re able to do things now that were impossible five years ago.” What are these things?
“With a data set that now comprises more than two million hours of its microbes at work, LanzaTech has trained machine-learning models that help scientists predict how their experiments will perform, thereby reducing the number of trials they need to run in the lab. ‘We build some microbes, we collect our data, and then we feed it into an AI system, which will then recommend what we should build next,’ Daniell said. LanzaTech is also exploring generative AI models. The technology is letting the company design DNA sequences for various enzymes, including some that allow microbes to turn carbon into isoprene, a raw material used for making rubber. LanzaTech then programs those sequences into its microbes for further testing.”
Reading, writing and recoding DNA using AI is becoming easier, and to talk about this some scientists and entrepreneurs are reusing the old metaphor of the book or language of life.
The language of life meets large language models
Since the 1960s scientists and then science popularisers have used the metaphor of the book of life for the human genome, a book written in the coded sequence of DNA using the four ‘letters’ ATCG (i.e. four bases: adenine, thymine, cytosine and guanine). The metaphor is basically as old as the discovery of the double helix itself. Around the year 2000, with the sequencing of the human genome, scientists’ ability to ‘read’ ‘the book of life’ increased massively, and some years later, around 2007, there was increasing talk about them being able to ‘write’ novel books of life or genomes themselves. They seemed to have cracked ‘the language of life’. After 2015, with the advent of gene editing, things began to accelerate. Now, with generative AI, we might be taking another big step forward.
In fact, the metaphors of book, language and code of life are turning literal, something that is not uncommon for metaphors. This has, for example, been observed for the metaphor of ‘cell factory’. As Andrew Reynolds has pointed out: “The metaphorical description of the cell as a factory became literal when scientists genetically engineered bacteria to express the gene for human insulin (among others), turning these cells into literal factories for the production of valuable commodities.” Let’s see how that literalisation works for the language of life.
AI learns to speak DNA
In the same WSJ article in which LanzaTech appeared, I also learned about another case of fusion between AI and synbio taking place at another company, Ginkgo Bioworks, which “uses tools such as robotics, software and data analytics to genetically program cells to produce compounds for applications in areas such as food ingredients, fragrances, cosmetics and medications.”
This is what Chief Executive Jason Kelly had to say about synbio and AI: “The same way that foundation models like OpenAI’s GPT are trained on the English language, they can ‘learn to speak DNA,’ Kelly said. ‘DNA is a sequential coded language, very similar to a book.’”
In an interview with CNBC, he elaborated at bit on this and said (roughly transcribed): “Instead of giving it books written in English, we give it books written in DNA…It’ll learn to speak DNA just like ChatGPT learned to speak English….. it will learn to write DNA better than a scientist doing it by hand”.
There are probably many more companies that want to build what many now call “DNA language models (also called genomic or nucleotide language models)”. Some people also talk about ‘protein language models’.
An example is ‘Ankh’ (ancient Egyptian symbol representing life) “created by a group of experts from the Universities of Munich and Columbia in collaboration with the biotech company Protinea”. Ankh “learns the ‘language of proteins’ by analyzing a large dataset of protein sequences, and then uses this knowledge to create new protein sequences and then attempts to determine how they might work.”
The article for Decrypt, entitled “AI could unlock the language of proteins” and talking about this new development in synbio, explains:
“In proteins, the alphabet consists of amino acids. These amino acids link together to form chains, sort of like words. The sequence of amino acids must be in a specific order for the protein to fold into the correct 3D shape, which is essential for its function. Basically, it’s like the way people put words together in a specific language, following a set of rules in order to properly communicate. A Large Language Model works by trying to predict which word would make the most sense in a specific output according to a prompt, and Ankh basically tries to do the same, guessing which biological configuration would make the most sense for a specific output considering everything we know about proteins and their structural rules.” (This is linked to the success of AlphaFold, something I can’t go into here, but it’s huge)
As pointed out in an article for Fierce Biotech, “Using the DNA of hundreds of people, as well as Cambridge-1, the most powerful supercomputer in the U.K., the researchers found it was feasible to develop a generalizable program—a ‘genomic language model’—that could be applied to a variety of different tasks, instead of requiring scientists to build fit-for-purpose AIs to chase answers for each major biological question.” (Italics added)
The possibilities are endless, it seems, when we move from the language of life to genomic language models, as the summary of an interesting article highlights: “ – DNA language models can easily identify statistical patterns in DNA sequences. – Applications range from predicting what different parts of the genome do to how genes interact with each other. – The hallucinatory tendencies of generative AI can be repurposed to design new proteins from scratch.”
And with gene interaction, we come not to the revitalisation of an old metaphor but to the death of another.
The dynamics of life and the death of the blueprint
There has recently been some discussion around the fact that the blueprint metaphor has become obsolete because it doesn’t capture the dynamics of living systems. Unlike the factory or the language metaphor, which seem to survive in new AI forms, the blueprint metaphor, interestingly doesn’t seem to survive the advent of new forms of AI and of new insights into the dynamic nature of life itself. As one article says:
As “AI enters a third wave, focusing on incorporating context into models, its potential to impact syn-bio increases. It is well known that an organism’s genotype is not so much a blueprint for a phenotype, but an initial condition in a complex, interconnected, dynamic system. Biologists have spent decades building and curating a large set of properties such as regulation, association, rate of change, and functions, to characterize this complex, dynamical system. Additional resources such as gene networks, known functional associations, protein-protein interactions, protein-metabolite interactions, and knowledge-driven dynamical models for transcription, translation, and interactions provide a rich set of resources to enrich AI models with context.”
Scientists are increasingly studying life in all its dynamic complexity and making use of new AI methods to help them do this better.
But…
This all sounds a bit too good to be true. We have to be careful when we are moving from predictive text to predictive DNA so to speak. All the problems we have with LLMs (large language models) might also affect GLMs (genomic language models), like ownership, copyright, exploitation of labour and many more. Some of the risks are beginning to be discussed for example in a new book entitled The Coming Wave: Technology, Power and the Twenty-First Century’s Greatest Dilemma written by Mustafa Suleyman and Michael Bhaskar … but more discussion and broader discussion is needed.
We have to be careful when reading statements that say for example: “The fusion of AI and Synthetic Biology is a game-changer. It’s not just about coding organisms; it’s about coding a brighter future.” Or indeed: “AI is turbocharging the frontiers of biological research, helping scientists program living organisms much as a software engineer might write code.” There is great stuff going on, but it’s sometimes good to take a step back and ask: where can this lead and whom will it affect, how, where and when? And: What language should I use to talk about this?
PS Just when I had finished writing this post on the 5 October, I saw this announcement: “The Wellcome Sanger Institute launches a new research programme that will combine large-scale genomic data generation with machine learning to predict the impacts of mutations and engineer biological systems….researchers will develop the technologies to write and edit genomes at scale and speed….to lay the foundations for predictive and programmable molecular biology”. This is called ‘Generative and Synthetic Genomics’…
Previous Post
Evelyn Fox Keller (1936-2023)Next Post
What are metaphors (for)?No comments yet, fill out a comment to be the first
Leave a Reply