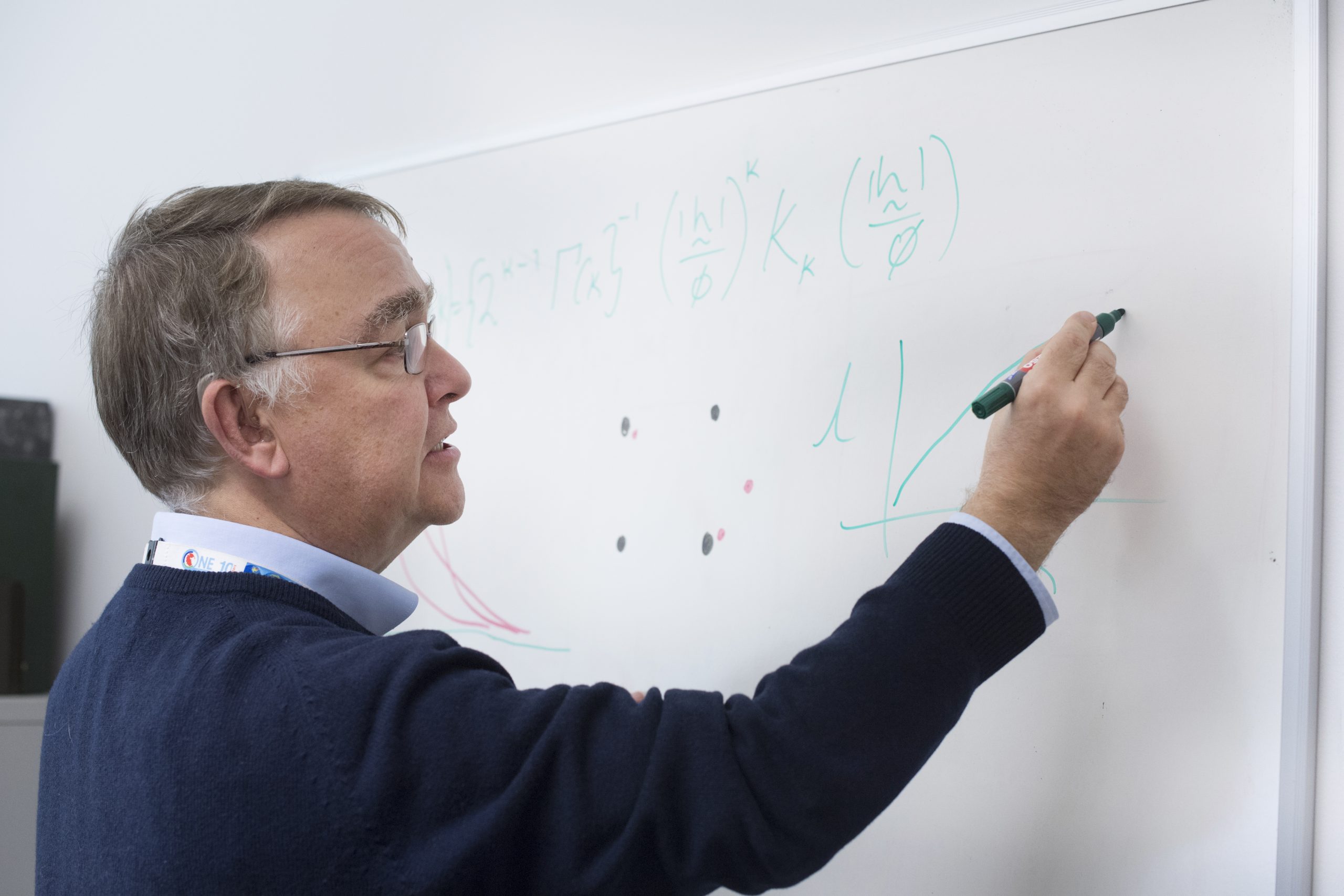
May 28, 2021, by Andrew Edwards (Ed)
Statistics in the GeoNutrition project – by Murray Lark, Christopher Chagumaira and Alice Milne
The intriguing message from our recent Nature paper (Gashu et al., 2021) is that the concentration of essential dietary micronutrients (like zinc and selenium) in locally-grown staple crops depends on where you look in the landscape. This was found in two African countries with contrasting cropping and food systems, but a shared common challenge of micronutrient deficiency in their populations. This message matters, because it means that a one-size-fits-all strategy to address micronutrient deficiency will be inefficient, and maybe ineffectual. The most appropriate intervention will vary from one location to another.
While the most obvious disciplinary contributions to this study are made by soil chemists, agronomists, plant scientists and nutritionists, we are glad for an opportunity to highlight the role of statistics. Statisticians are often regarded as number-crunchers to be called into a project right at the end to add a patina of respectability to the numerical outputs. That is not how it should be, and certainly not how it worked in the GeoNutrition project. Statisticians were involved in formulating project objectives, agreeing on the project’s broad approaches, and with every stage of implementation from planning the field work to writing the paper. We like to think that this is one of the reasons the project has been so successful.
How do statisticians contribute?
A key contribution from statistics is conceptual. The paper states in its title that nutrient concentrations vary “geospatially”, but what does that mean? Consider the two figures below: these are images with random numbers, from small values (white) to large values (dark green) each in a square array. You could think of them as representing the concentrations of micronutrients in a staple crop at a set of locations on a regular grid.

Fig.1a

Fig.1b
Now, the values in the two figures have the same mean, and vary about the mean to the same extent – their histograms are identical – but there is clearly a difference. In Fig. 1(a) you can see spatial structure, larger values tend to appear in distinct patches, as do small ones. In contrast, in Fig. 1(b), large and small values commonly occur next to each other. In Fig. 1(b) the values are independent, knowing the value at one location tells you nothing about the values at other locations. In Fig. 1(a) the values are spatially dependent; what this means is that neighbouring observations are, on average, more similar than observations further apart. Spatial dependence will arise when factors that operate at contrasting spatial scales contribute to variation (such as farm practices, soil, geology, and climate). It is this spatial dependence which is key to the GeoNutrition concept.
If micronutrient concentrations in grain are not spatially dependent, then this suggests that factors like soil and climate are not significant and there is no reason to expect the micronutrient supply from staples to differ systematically between one environment and another. Statisticians can use suitable models to quantify the evidence for spatial dependence from data, and to quantify the spatial scales which appear to dominate the variation.
Statistics also feeds directly into systematic planning of project work. A famous statistician once said: “To consult the statistician after an experiment is finished is often merely to ask [them] to conduct a post mortem examination. [They] can perhaps say what the experiment died of.”
An experimental or sampling design is fundamental to the analysis and inference which follows. We cannot analyse data unless we know how those particular locations or experimental units were selected. All too often, as the quotation above implies, the statistician is engaged only after the selection has happened, and then realizes that flaws in the approach mean that analysis is not possible, or will be much less powerful than it might have been.
Sampling design

Christopher Chagumaira leading a consultation with data-users in Addis Ababa
In GeoNutrition the sampling design was carefully selected by statisticians after discussion across the project team. The overriding objective was to support the investigation of spatial variation in micronutrient concentrations and their representation as a map. A sampling design was selected which would give good spatial coverage of the area of interest but also allow effective modelling of the spatial dependence. The design was discussed with the field sampling teams and adapted, as necessary, to deal with practical constraints, such as accessibility of sites.
Once the data are collected, the analysis must be consistent with the design. As the sampling design did not involve selecting target points independently and at random, this means that analyses cannot treat our observations as independent (as is done in much conventional statistical analysis) so we must account for possible spatial dependence.

Alice Milne explains how statistics is important in spatial mapping of soil to members of the public at Rothamsted Soil Research Day
This was done, for example, when fitting models to evaluate evidence that human biomarker data (which measure the evidence for micronutrient status) are related to concentrations of the micronutrient in locally-grown staple crops (see this figure from the paper), or that the concentration in the crop is related to soil properties (see this figure).
Spatial statistical models allowed us to produce the maps of grain nutrient concentrations shown in the paper (see here and here). The model allows us to make the best prediction of what the nutrient concentration would be at an unsampled site. It also allows us to quantify the uncertainty in that prediction, so that we can account for the degree of confidence in that prediction if we use it to make decisions.
In practice, a user of data might not be interested in point predictions, but rather in the mean values for administrative regions (for example, to decide on the amount of nutrient supplementation needed there, or nutrient-enriched fertilizer required). Again, statistical methods allow us to make the best possible estimates of the mean value for such regions, not just averaging the sample points from within those locations, but also referring to observations in neighbouring regions. Such maps for grain concentrations of zinc and calcium in the woredas (districts) of the Amhara region of Ethiopia are shown here in the paper.

Christopher Chagumaira (right) examines data-users’ responses to quantifications of uncertainty in spatial information
Always planning ahead
We hope that our colleagues in GeoNutrition agree that statisticians have proved their usefulness for such work! What of the future? One of us (Christopher Chagumaira) is completing a PhD project aligned to GeoNutrition. Among the problems he is addressing is how the information about the uncertainty of maps of crop nutrient concentration, which the statistical model can supply, can be most effectively communicated to users of that information so that they can make robust decisions which account for uncertainty. This has involved engaging with a variety of data users in Malawi and Ethiopia, and of course by planning carefully designed experiments to provide data for appropriate analyses. You can read some of Christopher’s conclusions here.
This is very insightful. I agree that statisticians are mostly engaged at end of a research. This needs to be changed