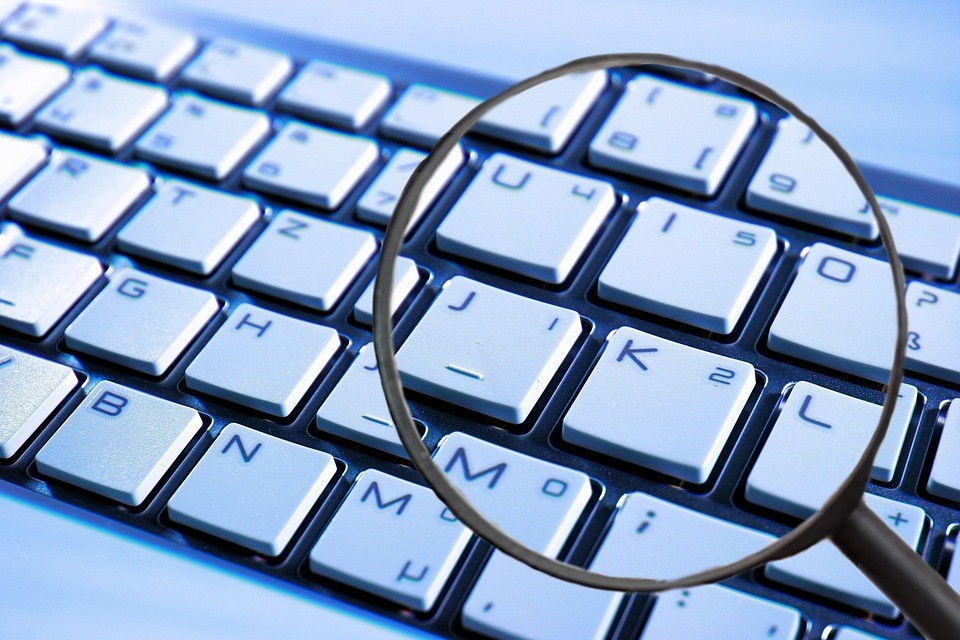
July 11, 2017, by Sunita Tailor
The finer details of the bigger picture: corpus linguistics in healthcare
This blog piece will introduce a relatively new method in the study of language – the corpus linguistic approach – and talk about how it can be useful for linguistic researchers interested in analysing communication in healthcare environments. To do this, this entry will ask – and answer – three questions: (i) What is corpus linguistics? (ii) What does a corpus linguistic analysis look like? and (iii) What can corpus linguistics offer to healthcare research?
What is corpus linguistics?
Corpus linguistics is a collection of methods that involve using specialist computer programs to study large amounts of texts or language data. This collection of language is known as a corpus (plural corpora) – the Latin for ‘body’. The corpora that corpus linguists analyse are usually very large in size, often amounting to millions, and occasionally billions, of words. The appeal of corpus methods, therefore, is that they allow us to analyse much larger and more representative amounts of data, using computer-aided methods that bring with them a degree of replicability and objectivity which can’t usually be achieved through purely manual analytical approaches.
What does a corpus linguistic analysis look like?
With the help of specialist computer programs (e.g. WordSmith Tools, AntConc), corpus linguists can quickly (and reliably) search for the most prominent linguistic patterns across their data. A useful technique for identifying salient patterns in a corpus is keywords. Simply put, keywords are words which occur significantly more often in one corpus compared with another. Keywords can therefore be considered to be characteristic of the corpus we are analysing (or more accurately, the genre or variety the corpus represents) and can flag up important themes in the data. As an example, the keywords below were generated from a 40 million-word corpus of patient feedback about the National Health Service (NHS) in England.[i] These keywords were generated by comparing this corpus against 1 million words of general British English.[ii]
Top 20 keywords from a corpus of patient feedback, ranked by LL[iii]
Rank | Keyword | Frequency | Keyness value (LL) |
1 | I | 985,701 | 36990.81 |
2 | of | 336,129 | 15889.81 |
3 | my | 362,005 | 15079.67 |
4 | staff | 159,892 | 10641.52 |
5 | appointment | 138,623 | 10205.98 |
6 | surgery | 134,415 | 10157.05 |
7 | his | 19,483 | 9997.14 |
8 | he | 37,513 | 9466.19 |
9 | very | 178,198 | 8503.30 |
10 | have | 331,258 | 8051.72 |
11 | doctor | 106,777 | 7425.61 |
12 | me | 193,232 | 6831.10 |
13 | dentist | 73,424 | 5636.02 |
14 | practice | 89,194 | 5577.85 |
15 | they | 253,491 | 5165.38 |
16 | care | 89,466 | 5062.46 |
17 | GP | 67,483 | 5002.82 |
18 | doctors | 66,364 | 4628.39 |
19 | service | 78,691 | 4177.62 |
20 | to | 946,728 | 3790.09 |
Although many of the keywords above (including the top 3) are grammatical words which don’t tell us much about the content of the patients’ comments, other keywords in this table are more revealing in terms of key themes in the data. For example, keywords like staff and dentist gesture towards the importance of staff members to the ways that patients provide feedback, while words like doctor, GP and doctors suggest a focus on doctors in particular. Other keywords reveal a focus on sites of care (surgery, practice), appointments (appointment), as well as more general concepts like care and service. Having identified key themes in the data, corpus linguistic techniques also allow us to then investigate more qualitatively how the patients actually talked about those themes in their comments.
Such a qualitative analysis typically involves exploring a particular keyword or set of keywords of interest by studying the words that tend to occur alongside it/them in the data. These words are known as collocates. By analysing a keyword in terms of its frequent collocates we can get a sense of how that word tends to be talked about in the texts in the corpus. To illustrate what a collocation analysis looks like, the table below shows the top 20 words which occur most frequently within the three words preceding and following the word appointment throughout the patients’ comments.
Top 20 collocates of appointment, ranked by LL
Rank | Collocate | Frequency | Number of comments | LL |
1 | an | 74,965 | 49,873 | 323085.77 |
2 | get | 27,090 | 21,784 | 80066.48 |
3 | book | 8,134 | 6,786 | 33985.88 |
4 | make | 9,467 | 7,838 | 29910.98 |
5 | for | 28,913 | 22,951 | 29714.26 |
6 | emergency | 4,676 | 4,084 | 13164.68 |
7 | system | 4,201 | 3,516 | 10622.48 |
8 | getting | 3,870 | 3,654 | 10080.61 |
9 | booked | 2,647 | 2,414 | 7669.61 |
10 | to | 41,969 | 30,539 | 7409.76 |
11 | booking | 2,180 | 2,035 | 6671.18 |
12 | day | 5,244 | 4,921 | 5793.62 |
13 | next | 3,079 | 2,867 | 5694.95 |
14 | cancelled | 1,379 | 1,167 | 4526.40 |
15 | my | 17,549 | 14,071 | 4285.79 |
16 | same | 3,081 | 2,942 | 4135.81 |
17 | another | 3,133 | 2,794 | 3645.12 |
18 | urgent | 1,512 | 1,391 | 3260.35 |
19 | offered | 1,498 | 1,423 | 3094.87 |
20 | weeks | 2,852 | 2,707 | 3091.29 |
Scanning this list of collocates, we might note that over a third of the words are to do with the process of getting an appointment (get, book, make, getting, booked, booking, offered). Other themes we might note are a focus on emergency appointments (emergency, urgent), appointment booking systems (system), cancellations (cancelled) and waiting times (weeks). While the collocates therefore provide a flavour of the kinds of appointment-related topics that the patients discuss in their feedback, to understand what it is that they are actually saying about these topics, we need to dig a little deeper and read a selection of comments in their entirety. We can do this by reading and analysing concordance lines. Concordance lines display all the instances of a word or phrase in the corpus with a few words of surrounding text, thus allowing us to inspect any recurring patterns of use within the comments more widely. Continuing with our focus on the theme of appointments, the six randomly-selected concordance lines below are of the phrase emergency appointment.
Concordance lines of the phrase emergency appointment
1 | in the last few months. And when I did need an | emergency appointment | I had to wait in all day for a doctor to call |
2 | seven to ten days minimum. You can try to get an | emergency appointment | , but you are cross examined by the receptionist and |
3 | always decide you do not meet the criteria for an | emergency appointment | . For appointments with a specific doctor, I have been |
4 | Very Helpful Doctors and Staff. Went for an | emergency appointment | and on a busy day got seen by a doctor relatively quick |
5 | takes a fortnight. If you want to make an | emergency appointment | they never have any. They claim to take bookings |
6 | is ridiculous, you can not get through, even for an | emergency appointment | . I was told to use the walk in centre in Dewsbury |
From this admittedly small sample of concordance lines, we can observe some interesting trends in comments about emergency appointments. First, these comments tend to be negative (five out of six are negative, only #4 appears to be providing positive feedback). These negative comments point to a series of patient concerns about emergency appointments, which include waiting times (#1, #2, #5), being cross-examined by receptionists and other medical gatekeepers (#2), not qualifying for an emergency appointment (#3), a lack of appointment availability (#5) and being unable to book emergency appointments over the phone (#6). Therefore, this small selection of comments suggests that most comments about emergency appointments are negative, with appointment access a key area of patient concern.
What can corpus linguistics offer to healthcare research?
Communication, and language in particular, plays a significant role in reflecting and shaping the ways that people think about and experience health and healthcare in a range of clinical contexts. Early linguistic research into health communication relied heavily on relatively small data sets more suited to granular, qualitative analyses, such as samples of language taken from face-to-face clinical encounters or research interviews. A criticism often directed at such research was that the findings presented were based on limited datasets that were not necessarily representative of wider communication within the particular clinical context of interest. More recently, corpus linguistic methods have helped researchers to overcome some of these barriers, affording the possibility to learn about the linguistic character of health-related communication by studying large amounts of data representing communication across a wide spectrum of clinical contexts. Moreover, with the help of quantitative computational measures like keywords and collocation, linguists are able to ground their analyses in more statistically-robust evidence, thus enabling them to provide insights that come closer to meeting the standards for evidence-based research which are presently commonplace in the world of scientific medicine.
Although I have focussed here on the language of patient feedback, the established techniques introduced are adaptable and have been applied to the study of: patient-practitioner encounters, first-person illness accounts, media reporting of health and illness, and the language of e-health and online advice-seeking, to offer just a few examples. As the short analysis described here has hopefully demonstrated, the combination of quantitative corpus methods with a more qualitative, human-led perspective on language can powerfully elucidate significant patterns and commonalities in any communicative context, generating insights which can greatly enrich our understanding of the ways in which people communicate about health and illness. And this combination – of computational methods and human-led analysis – is crucial to such analyses. To end with a caveat: though the computer can flag up frequent and statistically interesting patterns in the data, it is up to the human user to explore and explain why they are significant.
Dr Gavin Brookes
LiPP Research Fellow/Business Consultant
[i] This data originates from the Beyond the Checkbox project in the ESRC Centre for Corpus Approaches to Social Science (CASS) at Lancaster University.
[ii] The corpus used to represent general British English is the BE06 (Baker, 2009).
[iii] Keyness was measured using the log-likelihood (LL) (Dunning, 1993) statistical confidence measure. The higher the LL score a keyword is assigned by the computer, the greater confidence the researcher can have that that keyword is statistically significant.
No comments yet, fill out a comment to be the first
Leave a Reply